Just a day before the China Auto Chongqing Forum (CACS), Li Auto CEO Li Xiang made a spontaneous decision to change his speech focus from artificial intelligence to autonomous driving. During the forum, Li emphasized that future autonomous driving will emulate human capabilities, including quick response times and logical reasoning to handle complex scenarios.
It wasn’t clear at that time why Li decided on the last-minute change, but subsequent events offer some insight. A month later, Li Auto revealed an end-to-end system enhanced by a vision language model (VLM), which has become a hot topic in the smart driving industry.
Unlike its domestic rivals using a segmented approach, Li Auto’s solution resembles Tesla’s “One Model” design more closely.
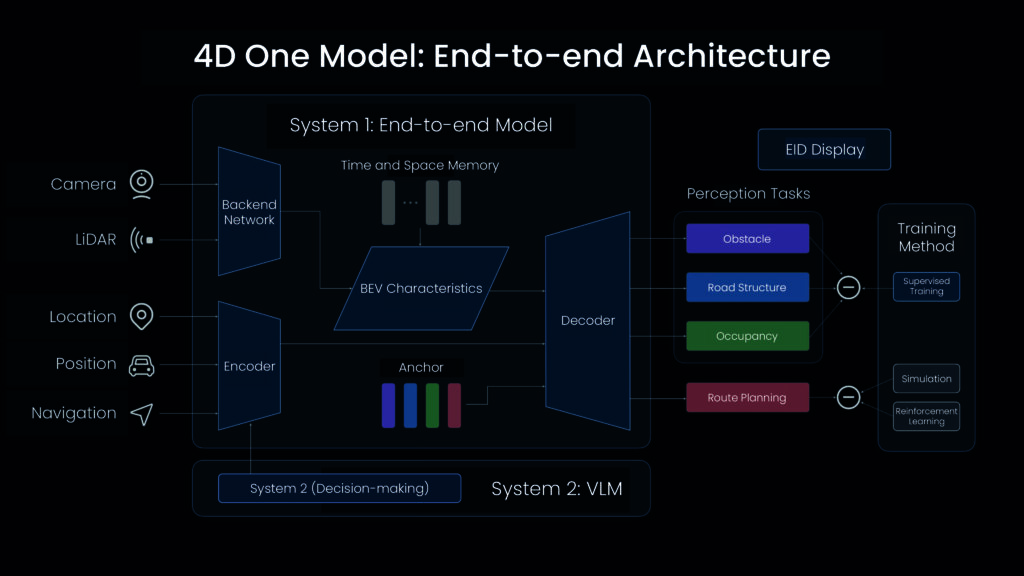
Historically seen as a follower in smart driving, Li Auto frequently shifted its approach last year amid fierce competition, first relying on high-definition maps, then lightweight maps, before discarding them altogether.
In a recent interview with 36Kr, Lang Xianpeng, vice president of smart driving R&D, and Jia Peng, head of smart driving technology R&D, discussed Li Auto’s ongoing journey. Reflecting on efforts to catch up, Lang surmised that the core principle is to identify the essence of the problem and then decisively and quickly correct the course.
Choosing the end-to-end (E2E) technical route is an extension of this principle. Lang explained that previous smart driving solutions have fundamentally been map-based. They followed the conventional process of managing control with perception, with upstream flaws requiring downstream adjustments, demanding significant investment.
However, the core issue isn’t just resource allocation but the fact that rule-based smart driving has a ceiling and is unlikely to fully emulate human driving.
Combining E2E with a VLM and what Li Auto calls a “world model” is the optimal paradigm that the automaker has now arrived upon.
In simple terms, Li Auto’s approach eliminates the previously separate modules for perception, prediction, planning, and control, merging them into a single neural network.
The VLM acts as a plugin similar to ChatGPT for the system. While the E2E system’s behavior depends on the data it receives, the VLM provides cognitive and logical reasoning capabilities. In complex scenarios, the system can query the VLM in real-time for driving assistance.
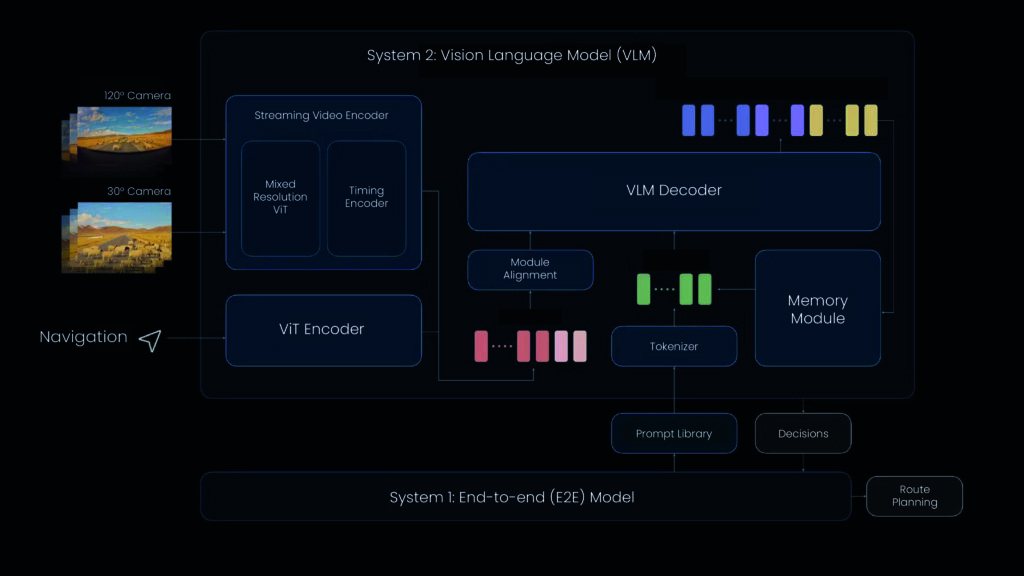
The world model serves as a massive problem set, generating simulated data through reconstruction and production methods, combined with real-world cases previously accumulated by Li Auto to create a mix of real and simulated test scenarios to challenge the E2E model. Only models that score well on these tests are released to users.
Internally, these three models are referred to as Systems 1, 2, and 3. System 1 corresponds to the brain’s immediate thought mode, System 2 to logical thinking, and System 3 acts as an examination model, assessing the learning outcomes of systems 1 and 2.
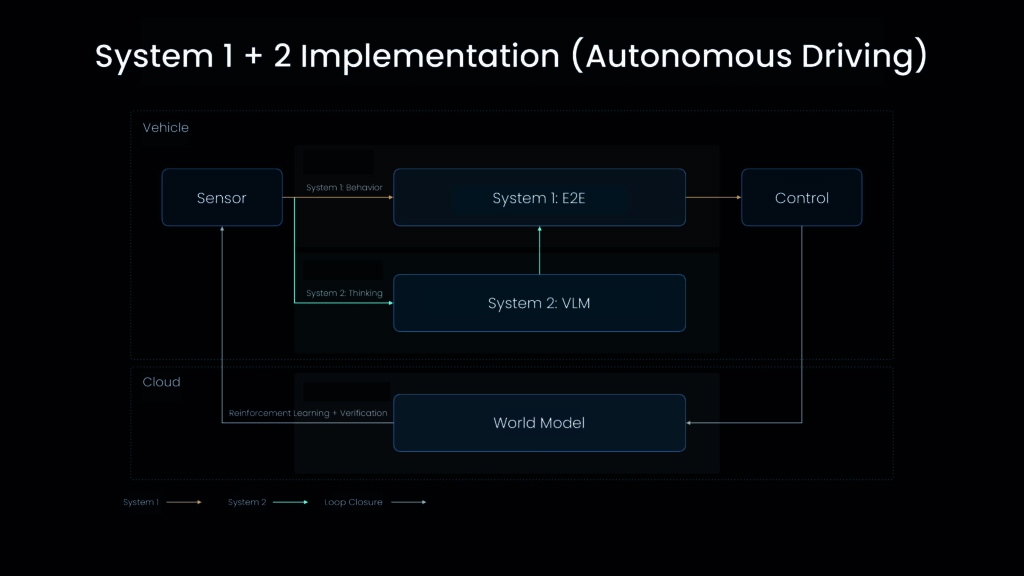
E2E smart driving technology was initiated by Tesla. In August 2023, CEO Elon Musk demonstrated the capabilities of its Full Self-Driving (FSD) tech in its V12 version during a live stream, which has now iterated to V12.5. However, unlike Tesla, Li Auto incorporates the VLM capability in addition to the E2E and world models.
Jia Peng explained to 36Kr that he spent a week each in the west and east of the US testing Tesla’s FSD and found that even E2E tech has limitations. In complex road conditions on the East Coast, such as New York and Boston, Tesla’s takeover rate significantly increased. Jia said that the parameters of the E2E model that can run on HW3.0 are not particularly large, and the model capacity has a natural upper limit.
Li Auto’s design of the VLM role aims to enhance the system’s upper limit. The VLM can learn from various scenarios, such as bumpy roads, schools, construction zones, and roundabouts, providing crucial decision-making support.
Lang and Jia both believe that the VLM is a significant variable in Li Auto’s smart driving system. With parameters already reaching 2.2 billion and a response time of 300 milliseconds, if equipped with more powerful chips, the VLM’s deployable parameters could reach hundreds of billions, marking the best path toward Level 3 and 4 autonomous driving.
“VLM itself is also following the development of large language models (LLMs), and no one can yet answer how large the parameter count will eventually be,” Jia said.
Based on the trajectory of data-driven, vision language models, it seems that the smart driving industry is now part of the computational power contest initiated by companies like OpenAI, Microsoft, and Tesla.
Lang candidly stated that, at this stage, the competition is all about the quantity and quality of data and computational power reserves. High-quality data relies on an absolute data scale— supporting Level 4 model training requires about tens of EFLOPS of computational power.
“No company without a net profit of USD 1 billion can afford future autonomous driving,” Lang asserted.
Currently, Li Auto’s cloud computing power is 4.5 EFLOPS, rapidly closing the gap with leading companies like Huawei. According to 36Kr, Li Auto has recently bought a large number of Nvidia’s cloud chips, purchasing almost all available stock from distributors.
CEO Li is well aware of this competitive landscape, leveraging resources and intelligent technology to outpace competitors. He often asks Lang if there’s sufficient computational power, and if not, to source more from Xie Yan, the company’s CTO.
With cars and more money than other players, Li Auto has an opportunity to widen the gap on this path. Financial reports show that, as of the first quarter of this year, Li Auto’s cash reserves are close to RMB 99 billion (USD 13.8 billion).
Data from Li Auto also indicates that the commercial loop of smart driving is starting to take shape. In early July, Li Auto began delivering the 6.0 smart driving version, which can operate nationwide, to Max model drivers. Lang observed that the proportion of Max models quickly surpassed 50%, with over 10% growth each month.
Lang also understands that, although the long-term vision of Level 4 autonomous driving is becoming clearer, its implementation path remains unchanged. “We need to quickly help the company sell cars. Only by selling cars can we afford to buy chips to train smart driving.”
If smart driving is the decisive factor in the automaking race, it represents a fiercely competitive and resource-intensive arena. Li Auto has proactively prepared by integrating top-level strategic planning, technical advancements, and substantial resource investments. But what about the others?
The insights above were derived from an interview conducted by 36Kr with Lang and Jia. The following transcript is a translation of that interview and has been edited and consolidated for brevity and clarity.
Tackling the limits of smart driving
36Kr: Have you reviewed internally how Li Auto managed to reach a level comparable to Huawei from a lagging position in smart driving?
Lang Xianpeng (LX): Compared to Xpeng Motors, Nio, and Huawei, it’s not that we have more brains. In fact, we might even have fewer people, but our focus is on being pragmatic. Sometimes, people miss the essence of the problem and merely tweak what’s currently being done without addressing the core issues.
Take the example of using maps: the map itself often poses the biggest problem. Initially, we invested a lot of effort into working with maps. However, once we identified the core issues associated with map usage, we quickly corrected our course and shifted our investment to the next phase of research and development.
36Kr: How did you correct the course for nationwide mapless smart driving, which has seen many versions?
LX: At the Shanghai Auto Show last year, the focus shifted to developing urban navigate-on-autopilot (NOA) solutions. Initially, the plan was to adapt high-definition maps, used for highways, for city use. We consulted AutoNavi, which had high-definition maps for about 20 cities, and decided to proceed.
However, the iteration and update of plans and maps were closely intertwined. When we were working in Wangjing, road repairs, detours, and even traffic light changes required us to wait for AutoNavi to update the map before continuing work. Around June last year, we decided to stop relying on these heavy maps and switched to the neural prior net (NPN) approach. This method uses local mapping at major intersections and roundabouts, with our vehicles updating the features in real-time.
But in smaller cities with fewer cars, how would updates happen? Would we always work in big cities? Users wouldn’t buy that. Internally, there were still hesitations. Some voices suggested sticking to a few first-tier cities, as Huawei initially did with 50 cities.
I said no, we still need to move quickly and see if the NPN method is feasible on a larger scale. The issue with maps is their inherent limitations, and some cities are criticized for having only two operational routes. Therefore, we decided to switch to a mapless approach after expanding our coverage to 100 cities in December last year.
36Kr: What was needed to move from mapless navigate-on-autopilot (NOA) to an end-to-end (E2E) system?
LX: There are still issues with going mapless. Previously, maps provided accurate information, but without map priors, the demands on upstream perception become exceptionally high. Any perception-related jitter issues make downstream control significantly challenging.
Continuing down this path required a large workforce. For example, if perception had issues, we had to add numerous rules to the middle environment model. If downstream control was affected, we had to add compensatory rules. This posed a significant human resource challenge. Huawei’s mapless approach leverages their manpower advantage, and we also considered increasing our workforce last year.
But there is a clear upper limit since all rules are human-made, relying on engineers’ designs. Earlier this year, we often found that changing one rule would fix one case but break another. The interdependencies were vast and endless.
Resource allocation isn’t the main issue; the key problem is that rule-based experiences have a ceiling and can never truly emulate human driving. Hence, we iterated to the current E2E and VLM approach. E2E marks the first use of AI in smart driving.
36Kr: When did Li Auto start investing in E2E technology?
LX: We always have two lines of work.
The first one’s visible and targets mass production. Last year, this was to transition from NPN lightweight maps to mapless.
The other, which is shielded from the public eye, is for preliminary research, focusing primarily on E2E technology.
It was only made explicit during the strategic meeting at Yanqi Lake last year. At the strategy meeting, Li Xiang emphasized that autonomous driving is our core strategy, and R&D must achieve important milestones. The E2E concept had been there for a while but lacked resources for exploration due to delivery pressures.
36Kr: Li Auto may need to transit from mapless to E2E soon. How do you plan the pace of this transition?
LX: At the beginning of the year, I told Li Xiang that although our goal is to achieve E2E solutions, we still need to deliver mapless technology first, as it supports E2E. Without it, where would the data and experience come from?
Moreover, mapless technology must be launched first to sell cars and compete with Huawei. Now that it is launched, it buys time for E2E development while enhancing our product features to aid car sales.
36Kr: Your plans have continuously changed in this process of correcting the course. Is there pressure from upper management?
LX: No, my responsibility is to lead the team in achieving autonomous driving. At Li Auto, we have our own methodology and process. Doing the right but difficult thing might sound cliché, but it’s crucial.
Li Xiang never questions why I negate previous efforts. Once we explain why a particular approach is necessary, especially for winning in AI strategy and finding a dual-system paradigm, he immediately understands. He would only say that E2E is great, and to do it quickly.”
AI requires computational power and data. Li Xiang often asks me if we have enough computational power, and if not, to get more from Xie Yan.
Li Xiang also emphasizes that we have cars and more money than others, giving us a great opportunity to widen the gap. So, let’s not waste time on patchwork—focus on AI.
Regarding the future of smart driving
36Kr: Some companies believe E2E is a chance to overtake competitors without having explored mapless solutions. Is this a valid perspective?
LX: They are half right. E2E can indeed switch tracks. Whether it’s with maps, NPN, or mapless, the core is the same. Removing the map enhances perception, and small modules evolve into larger models using a unified solution step by step.
But E2E is different. It’s the first time AI is used for autonomous driving. With the One Model, the input is only data, and the output is a trajectory, integrating all intermediate modules into one model.
The entire development process is different. Traditional product development is driven by demand design or problem feedback. In this scenario, if something doesn’t work, it undergoes manual design iteration and validation.
E2E is a black box where its capabilities depend entirely on the data provided. Currently, we filter data from experienced drivers. If the data is poor, the model will be poor. Garbage in, garbage out. […] Previously, it was a product function development process—now, it’s a capability enhancement process.
So, E2E can change tracks, but overtaking requires data and computational power. Without these, the best model is just a bunch of parameters.
36Kr: Li Auto has a lot of data, but He Xiaopeng recently suggested that more data doesn’t necessarily mean achieving autonomous driving. What’s your view?
LX: Our training data consists of clips, each comprising several seconds of driving data such as visual sensor data, vehicle status information, throttle, brake actions, and more.
However, for data to be useful, it must be high quality.
What constitutes high-quality data? We’ve defined what is the standard of a high-quality human driver based on product and vehicle performance evaluations conducted subjectively by our teams. Even highly skilled drivers aren’t ideal if they frequently accelerate or decelerate sharply or trigger autonomous emergency braking (AEB) or sudden steering.
According to these standards, only 3% of our 800,000 owners qualify. Combined with previously accumulated high-quality data, we have amassed millions of clips—all premium data. He Xiaopeng is correct that high-quality data is essential, but its quality is based on an absolute scale.
36Kr: After implementing E2E, does the data toolchain need upgrading?
LX: The toolchain has changed significantly. Previously, we manually analyzed user takeover data, modified code, and tested it in real vehicles. This process, while efficient, took several days and required significant manpower. More testing revealed more issues, necessitating more personnel for modifications.
Now, when a driver takes over, the data returns automatically and generates similar scenarios using the world model, forming a problem set. If there’s no similar data, we mine the existing database for joint training.
The new model undergoes two tests in the world model system: first, whether it corrects the initial error, and second, a set of test questions. If both are successful, the model is released. Ideally, this process involves no human intervention, creating an automated closed-loop.
36Kr: The E2E black box training process requires many fallback codes. Can you estimate the fallback workload?
LX: Very little. The map version had about 2 million lines of code, the mapless version had 1.2 million, and E2E has only 200,000—just 10% of the original.
For control, we do use some fallback rules. Since E2E inputs sensor data to generate trajectory, we add some brute-force rules to prevent extreme control behaviors, like turning the steering wheel 180 degrees.
36Kr: Elon Musk said he deleted 300,000 lines of code, and you seem even more aggressive. Will you add code back if more issues arise?
LX: I don’t think there will be much change. We continually iterate our capabilities.
36Kr: Li Auto is focusing on two areas: mass production and preliminary research. What’s the current research status?
LX: Level 4. This relates to our understanding of AI. We found that achieving true autonomous driving differs from current methods. End-to-end behavior depends on the data it receives. However, humans, for example, can drive in Beijing and also in the US. To achieve autonomous driving, the system must similarly understand and infer situations.
We studied how the human brain works and thinks. Last August and September, Jia Peng and Zhang Kun came across the dual-system theory, an excellent framework for human thinking. Assuming AI is dual-system, System 1 has quick response capabilities, and System 2 has logical reasoning abilities for handling unknown situations. For autonomous driving, the end-to-end model is System 1, and System 2 is the VLM. This is the ideal real-world implementation of AI.
How do we measure System 1 and System 2? We have a world model, internally called System 3, which acts as an examiner. We have a real question bank, real driving data, and the world model generates similar scenarios from existing data. When a model is trained, it first takes a real test and then several simulated tests. Each model has a score, and higher scores indicate stronger capabilities.
36Kr: When does System 2 get triggered?
LX: Systems 1 and 2 always work. In complex scenarios, System 1 may struggle, such as with overpasses, potholes, or freshly paved roads, where System 2 steps in. It works at a lower frequency, like 3–4 Hz, while System 1 runs at over 10 Hz, frequently asking System 2 for advice.
36Kr: Does the VLM have an upper limit in System 2?
LX: It can be viewed as a LLM, with varying abilities. We focus on driving-related regulations, driving test sections 1–4, teaching videos, and materials. Our VLM is essentially a driving-focused LLM.
Short-term, it may lack some knowledge, but as the closed-loop cycles increase, its capabilities will rise.
Currently, the E2E model has over 300 million parameters, while the VLM system has 2.2 billion.
36Kr: So, the larger variable in the future of smart driving is System 2?
LX: System 1 is the foundation, but advanced autonomous driving (Level 3 and 4) requires a powerful System 2. The current 2.2 billion parameters may not be enough—more will be added.
Jia Peng (JP): System 2 mainly handles complex scenarios, with a response time of 300 milliseconds for the 2.2 billion parameters, which is adequate. But System 1 needs a faster response, around tens of milliseconds.
36Kr: Will model parameters have an upper limit? For example, 8 billion? What are the chip’s computational power requirements?
JP: Similar to LLMs, no one can predict the upper parameter limit.
LX: We now have both theory and implementation. System 1 plus System 2 is a great AI paradigm, but realizing it requires further exploration.
36Kr: To evolve from E2E segmentation into the “One Model,” do you need to start over?
JP: It’s challenging. Our mapless solution is segmented, with just two models. First, the technical challenge of scrapping the traditional approach is significant. Second, the human challenge of combining perception and regulation teams with different backgrounds to develop the model is also notable.
Our team has struggled. In the E2E model, many roles change. Engineers may redefine data or scenarios. Adapting roles is challenging.
Closing the loop on commercialization
36Kr: E2E sounds costly. How much does Li Auto plan to invest?
LX: Definitely. Currently, it’s RMB 1 billion (USD 139.7 million). Future autonomous driving model training might require USD 1 billion, excluding other expenses like hardware and electricity. Companies without USD 1 billion in net profit can’t afford it.
36Kr: E2E might be a watershed technology for the automotive industry. How is smart driving performing commercially?
LX: Since version 6.0 launched, AD Max has exceeded 50% of orders, with over 10% monthly growth. In Beijing, Shanghai, Guangzhou, and Shenzhen, the ratio has reached 70%. [AD Max orders for the L9, L8, and L7 are 75%, 55%, and 65%, respectively.]
JP: The L6 model also holds 22%. For young buyers, smart driving is significant. Once experienced, it’s hard to go back.
LX: Highway NOA is widely accepted. City NOA is still in its early stages, with product strength not yet optimal. End-to-end will change this, bringing performance closer to human driving. With more data and computational power, smart driving in cities based on end-to-end architecture might match the highway driving experience. This will significantly impact car purchases.
36Kr: With smart driving’s growing commercial value, will Li Auto reconsider its free functionality strategy to highlight this value?
LX:Many buy Li Auto for the fridge, television, and large sofa, but future buyers might choose us for smart driving. This shows its commercial value. The difference between Max and Pro versions is just RMB 30,000 (USD 4,190).
As for software fees, if significant Level 4 capabilities can be achieved, imagine cars picking up kids from school. Wouldn’t you pay for this service? Additional business models will emerge with enhanced capabilities, but significant smart driving improvements are essential first.
36Kr: He Xiaopeng mentioned achieving a Waymo-like experience in 18 months. Do you have a timeline?
LX: If data and business support the goal, it’s achievable. We estimate that supporting VLM and end-to-end training requires tens of EFLOPS cloud computing power.
Xpeng has 2.51 EFLOPS, Li Auto has 4.5 EFLOPS, and at least 10 EFLOPS is needed to achieve the goal, costing about USD 1 billion annually. If we can afford it annually, we can participate.
36Kr: Besides computational power, what’s the annual average investment required for a smart driving team?
LX: Major expenses are training chips, data storage, and traffic, costing at least USD 1–2 billion annually. World model development, aiming to replicate the real physical world, also requires extensive training and computational power.
I can’t imagine the upper limit, but it’s at least tens of EFLOPS. Musk’s estimate of hundreds of EFLOPS isn’t farfetched.
36Kr: With automakers still operating on manufacturing profit models and price wars affecting profits, is it suitable for automakers to undertake the workings of tech companies?
LX: Companies with high-quality data, sufficient training computational power, and adequate talent can achieve great models. Besides Li Auto, Huawei, and Tesla, I can’t think of others.
Our goal is to help the company sell cars quickly, as selling cars funds smart driving training.
Smart driving development will widen the gap further. Previous map-based or mapless approaches aimed at visible ceilings. Further breakthroughs require AI, leveraging data and computational power. Those who can’t address this will remain stuck, while we move to the next dimension to capitalize on data benefits.
36Kr: How many personnel are required for E2E model development? What’s the future average size of the smart driving team?
JP: Not many. Tesla has about 20 elite model developers in its vision team. We can infer from OrinX chip parameters running at 12–15 Hz to determine model size and structure, requiring only a few people to define.
LX: Tesla is extreme, with 200 software algorithm personnel, but they only develop one chip and a few models. We aren’t as extreme but still need several times more people due to our diverse chip platforms and models, though not many overall.
36Kr: How will smart driving chips developed in-house integrate with the E2E model?
JP: Hardware-software integration will enhance performance, as Tesla has shown. They achieve cheaper chips, higher computational power, and better AD support, enabling them to increase parameters fivefold in FSD V12.5. This offers a significant advantage.
LX: The premise is that Level 3 and 4 algorithms must be established.
36Kr: Is there a timeline for Level 4 autonomous driving?
LX: In 3–5 years. First, we’ll deliver Level 3 [autonomous driving], which paves the way for Level 4. Level 3 helps us understand Level 4’s computational and data requirements, including exam systems and data loop fundamentals.
Second, we need to build trust with users as E2E is a black box, and people may not fully trust the system. Level 3 products help establish this trust.
36Kr: Following Tesla, how does Li Auto ensure accurate tech judgment and avoid missteps?
LX: We have a complete system. While Level 4 requires 3–5 years, we’re exploring it now. If mistakes are made, it’s better to err early, with opportunities for correction.
AI divergence between China and the US is real, but the former has ample talent. We aim to recruit the best, and this year, we hired over 240 graduates from QS top 100 universities.
Learning from and surpassing Tesla
36Kr: Some say the gap between Chinese companies and Tesla in smart driving is two years. What’s your view?
LX: Definitely not. We don’t comment on technical solutions, as Tesla hasn’t disclosed much recently. From a product experience perspective, we’re at the level Tesla was when it released its E2E version last year—about a six-month gap.
36Kr: Tesla has faced issues like lesser data and feedback. How does Li Auto avoid them?
LX: Different stages signal progress to the next level.
JP: Tesla’s biggest issue now is validation. FSD V12.4 performed poorly, leading to V12.5’s fivefold parameter increase. I suspect validation was lacking, not knowing how it performs for users.
This is why we emphasize world model validation. We learned from Tesla’s challenges, ensuring comprehensive testing before nationwide rollout, including residential and community roads.
Tesla AI Day 2022 showed traditional simulation with poor scalability, unable to support full deployment across North America. We focused on world model development to address this.
36Kr: What challenges have you faced in E2E development, such as with the data toolchain?
JP: We’ve been building the data toolchain since 2019, [and are] likely the best in China. Data and training follow standard paradigms. Validation remains the biggest challenge.
Another is the evolving role of VLM. Initially used in 5% of cases, but as E2E reaches its limits, VLM handles the rest, posing future challenges.
This differs from Tesla. We developed VLM and world models to address Tesla’s issues. After testing in the US, we found FSD performed well on the West Coast but poorly on the East Coast, especially in complex cities like Boston and New York, with high takeover rates.
These insights led us to develop VLM, aiming to break this ceiling. VLM’s high potential offers a path to surpass Tesla.
KrASIA Connection features translated and adapted content that was originally published by 36Kr. This article was written by Li Anqi for 36Kr.