Amid ongoing debates about the limitations imposed by scaling laws on large models, the industry’s drive to innovate has never been more pronounced. In just the first month of 2025, major players in the large model space, including OpenAI, Google, DeepSeek, and others, have unveiled a flurry of new products, signaling an intensified race to meet performance targets.
For the first time, the large model industry is grappling with significant internal divergence and a lack of consensus on key issues:
- Application versus technology: Has foundational model development reached a plateau, prompting a shift in focus toward applications?
- Price versus value: Should companies engage in price wars? If so, how? Can startups remain competitive in this landscape?
- Unimodal versus multimodal: How crucial is multimodality in the pursuit of artificial general intelligence (AGI)?
At this critical juncture, every company in the large model industry is being compelled to take a stance.
For instance, OpenAI’s GPT-o1 seeks to extend the limitations of scaling laws through reinforcement learning, while Google’s Titans explores innovative memory architectures. Meanwhile, some companies are pivoting toward application optimization, feature enhancements, and improving user retention.
As one of China’s six “AI dragons,” MiniMax has made its position clear through a series of open-source releases and updates. Known for its strong product development, the company has shifted its focus toward innovation and open-source leadership.
In just ten days this month, MiniMax introduced four artificial intelligence models: the foundational language model MiniMax-Text-01, the multimodal visual model MiniMax-VL-01, the video model S2V-01, and the speech model T2A-01. Most notably, the MiniMax-01 series marks the company’s first step in open-sourcing its models.
In a recent interview, MiniMax founder Yan Junjie reflected on this strategic shift: “If we had to start over, we would have gone open source on day one.” In the industry, it is common for commercial companies to shift from open source to closed source, as exemplified by OpenAI. However, the reverse—transitioning from closed source to open source—is far less common.
With this wave of updates, MiniMax aims to redefine its market identity, evolving from a company known primarily for product strength to one recognized for its innovation, commitment to open source, and technological leadership. “Building a technology brand is essential because the greatest driver of this industry is technological evolution,” Yan said.
Confronted with the industry’s three major points of divergence, MiniMax is positioning these updates as its answers to the pressing challenges of the large model space.
Application versus technology
Since 2024, the large model industry has exhibited a notable trend: breakthroughs in foundational technology have slowed significantly.
OpenAI’s much-anticipated GPT-5 has faced repeated delays, with no release in sight. The three pillars of AI—computing power, algorithms, and data—are all experiencing varying degrees of stagnation.
In stark contrast, a fierce advertising battle over model applications has erupted. According to data from AppGrowing, the top ten large model apps in China have collectively run over 6.25 million advertisements since Moonshot AI entered the fray with heavy ad spending. Based on market rates, total ad expenditures have reached approximately RMB 1.5 billion (USD 210 million). Some industry observers have quipped that platforms like Bilibili, Douyin, and Xiaohongshu—the ad platforms—are the biggest beneficiaries of the large model industry.
At the application level, many companies are focusing on app products, custom collaboration projects, and developing smaller models tailored for government and enterprise use. Meanwhile, domestic and global players have largely adopted the conservative strategy of benchmarking OpenAI’s GPT in their technological roadmaps. However, with OpenAI seemingly hitting a plateau, the entire industry’s momentum appears to be slowing.
Amid this backdrop, MiniMax made waves on January 15 by releasing and open-sourcing its latest MiniMax-01 series. This release includes the foundational language model MiniMax-Text-01 and the multimodal visual model MiniMax-VL-01.
Accompanying the launch, a 68-page technical paper titled “MiniMax-01: Scaling Foundation Models with Lightning Attention” was published, sparking widespread discussion in research communities.
Technically, the MiniMax-01 series boasts 456 billion parameters and delivers performance on par with state-of-the-art models like GPT-4o and Claude-3.5-Sonnet across multiple mainstream evaluation datasets. It supports inputs of up to four million tokens—32 times more than GPT-4o and 20 times more than Claude-3.5-Sonnet.
In the latest LongBench V2 evaluation, MiniMax-Text-01 secured the third highest score, trailing only OpenAI’s o1-preview model and human performance. However, its robust performance isn’t the only aspect capturing the attention of AI researchers.
Notably, MiniMax’s introduction of “linear attention” marks a groundbreaking departure from traditional transformer architecture. This innovation is said to drastically reduce computational costs while addressing critical challenges facing the entire industry.
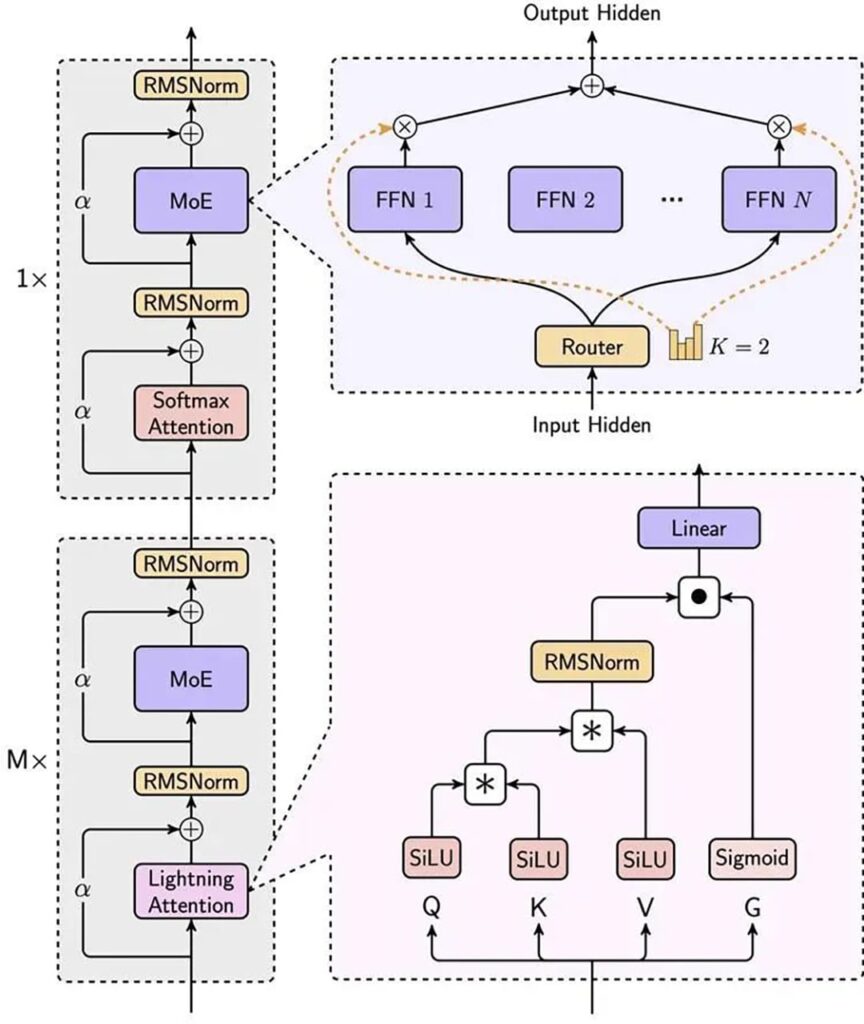
By reconstructing the core transformer architecture, MiniMax-01 embeds linear attention at its foundation, fundamentally altering the legacy structure of the technology. While linear attention mechanisms have been proposed before, MiniMax-01 is believed to be the first to implement them at scale. This breakthrough enables the model to deliver top-tier performance at just one-tenth the computational cost of GPT-4o. Additionally, its unprecedented four-million-token context length reportedly sets a new international standard.
In its accompanying technical paper, MiniMax disclosed that the MiniMax-01 series still relies on traditional transformer methods for approximately one-eighth of its architecture. The company is actively developing a new framework to eliminate these legacy components entirely, aiming to achieve an unrestricted context window.
If successful, MiniMax’s advancements could remove current input-length limitations in large models, potentially bringing humanity a step closer to realizing AGI.
Price versus value
If there was a defining trend for the large model industry in 2024, it would likely be the price war that has only intensified since. This competition has been most fierce in the B2B sector, particularly among providers of API services for large models, who charge customers based on token usage.
In May 2024, Chinese startup DeepSeek shocked the industry by slashing API prices for its DeepSeek-V2 model to as low as RMB 1 (USD 0.14) per million tokens—just a fraction of GPT-4 Turbo’s cost at the time. This move ignited a fierce price war, prompting rapid responses from companies like ByteDance, Baidu, Alibaba, Tencent, Zhipu AI, and iFlytek.
Since ChatGPT went viral in late 2022, the demand for Nvidia GPU chips—already in short supply—has skyrocketed, driving up prices dramatically. This surge pushed Nvidia’s market capitalization past USD 3 trillion, surpassing Apple to become the second most valuable company in the world after Microsoft. GPUs not only come with exorbitant costs but are also increasingly scarce. In 2023, an overseas AI startup reportedly secured USD 2.3 billion in financing by using Nvidia GPUs as collateral.
Caught between escalating computing costs and cutthroat price competition, large model companies found themselves in a difficult bind. However, as in many tech challenges, the solution lies in innovation.
For companies navigating the dual pressures of high computing expenses and relentless price wars, technical optimization has emerged as the key to survival.
Take DeepSeek as an example. Like MiniMax, DeepSeek prioritizes technology-driven solutions. In 2024, its V3 model scaled to 671 billion parameters, achieving a training cost of just USD 5.576 million. This was astonishingly lower than GPT-3’s training cost of nearly USD 12 million in 2020 and GPT-4’s cost exceeding USD 100 million.
Reducing training costs requires more than advanced algorithms—it demands comprehensive optimization across the entire infrastructure stack. This includes improvements in algorithms, architectures, hardware, software, and toolchains, collectively referred to as AI infrastructure. Amid soaring computing costs, the main goal of AI infrastructure is to maximize computational efficiency while minimizing deployment expenses.
MiniMax-01 exemplifies this principle with groundbreaking innovations like Linear Attention, which reduces matrix input complexity to cut computational costs significantly. Beyond this, MiniMax incorporates a range of optimizations across its data, algorithms, and GPU communication processes. These include data packing to improve input efficiency, linear attention sequence parallelism (LASP+) to enhance computational throughput, and multi-level padding to optimize memory usage.
These techniques enable MiniMax-01 to reach 75% model FLOPS utilization (MFU) on Nvidia H20 GPUs, reducing the costs associated with both training and inference.
When asked about MiniMax’s most gratifying technical milestone over the past year, founder Yan pointed to two key achievements: advancements in AI infrastructure and computational optimization, alongside breakthroughs in multimodal technology.
Unimodal versus multimodal
Among the many debates in the AI community, multimodality is arguably the least contentious—though the race to innovate in this space remains fierce.
In computing, a modality refers to a mode of interaction or perception between a computer and a user, such as text, images, audio, or video. Today, most major AI companies are heavily investing in multimodal models. While simpler models focus on text and images, advanced versions delve into audio, video, and even 3D modeling.
For instance, MiniMax’s T2A-01 speech model, launched on January 20, supports 17 languages and is now integrated into its Hailuo AI platform, which is accessible to all users. A demonstration video highlights the model’s synthetic speech capabilities, beginning at the 16-second mark. Listeners can identify speakers’ gender, age, and emotions without watching the visuals. Elderly voices convey frailty, women sound serious and resolute, teenagers express anger, and children’s tones are innocent and joyful. The emotional depth and cadence are strikingly human-like.
Video demonstrating the audio synthesis capabilities of MiniMax’s T2A-01 model. Video source: MiniMax.
Although text-to-speech technology has existed for years, early implementations were often rigid and mechanical. Google Translate’s famously robotic voice even became an internet meme. MiniMax’s model, however, has achieved commercial-grade quality, making it suitable for applications like AI audiobooks, radio dramas, animation, and video voiceovers.
Even more buzzworthy than its speech model is MiniMax’s video generation breakthrough.
In February 2024, the company unveiled its video generation model, Sora, sparking global excitement. However, while competitors rushed to release their own models, Sora remained in development until its eventual launch in December 2024.
The current landscape of AI video generation is divided into two main approaches, text-to-video and image-to-video:
- Text-to-video relies on diffusion technology, enabling creative content generation from user prompts. However, this method demands significant computational power, and results can be unstable—particularly with character faces.
- Image-to-video, which generates videos based on reference images, offers greater subject stability and lower computational costs, though it sacrifices creativity and flexibility.
MiniMax’s latest video model, S2V-01, released on January 10, bridges the gap between existing approaches to video generation. Its proprietary subject reference technology allows users to upload a reference image to extract subject information and generate videos based on textual prompts. This innovation ensures subject stability while preserving creative flexibility.
Video generated using S2V-01, MiniMax’s latest video model.
For example, one user uploaded an image of a man in a police uniform and entered the prompt: “A male police officer exits a police car. The camera follows him closely, focusing on his face. His expression changes from calm to aggressive. The cityscape is enveloped in night, with flashing police lights nearby.”
Video generated by MiniMax’s Hailuo AI.
Another user created a dystopian scene with the prompt: “Kowloon Walled City in a dystopian wasteland. A retired soldier walks cautiously down the street with a dog, avoiding patrolling drones flying overhead. Gunfire between mantis-like robots and resistance fighters echoes in the distance.”
As demonstrated in these examples, S2V-01 ensures facial details and features—such as moles or freckles—remain consistent across all frames. This capability could represent a breakthrough in AI video generation models.
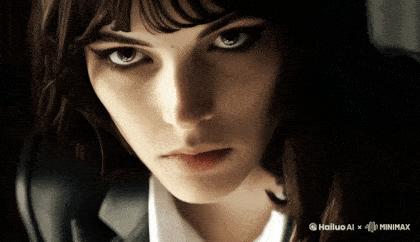
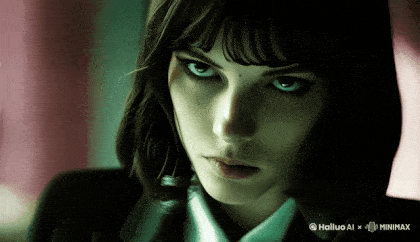
According to MiniMax researchers, S2V-01 processes text and visual information in ways that closely resemble how humans perceive and interpret multimodal inputs.
Multimodality, alongside multitasking and multi-contextual understanding, is a critical milestone in the pursuit of AGI. While some researchers argue that language models alone may achieve AGI, others see multimodality as essential. In the absence of consensus, agentic AI—systems capable of autonomous, goal-directed actions—has emerged as a more tangible and immediate goal.
As foundational technologies advance, industries increasingly demand AI agents that can handle complex tasks, process vast datasets, and function with heightened contextual awareness. Models like MiniMax-01 are at the forefront of this movement, offering extended context capabilities and human-like multimodal processing.
The year 2025 may well mark the rise of AI agents. By December 2024, MiniMax’s AI content platform, Talkie, had reportedly reached 29.77 million monthly active users, potentially making it the world’s largest AI content platform.
Given its leadership in the application space, one might expect MiniMax to fully embrace an application-first strategy. Yet, founder Yan recently challenged this notion in an interview, stating:
“The Chinese AI industry has fallen into a massive misconception over the past two years: the belief that more users equals faster model improvement. As a result, companies are spending enormous sums to buy user traffic.”
Frankly, he might be right.
While many large model companies around the world were founded after ChatGPT went viral in 2022, MiniMax was established earlier, in 2021. When ChatGPT’s sudden rise stunned the world, industry insiders were scrambling to understand who MiniMax was and how it managed to stay ahead of the curve.
In fact, MiniMax has consistently taken an unconventional approach to technology.
In 2023, the company allocated over 80% of its computational resources to developing mixture-of-experts (MoE) models. By early 2024, it introduced China’s first MoE-based large model. MiniMax has stated that it committed fully to this approach without fallback options, reflecting its confidence in the MoE pathway.
This decision has since aligned with broader industry trends, as the MoE approach has gained acceptance in addressing the challenges of scaling model sizes and computational demands.
Advancements in model capabilities and processing speed have also consistently improved product quality and user experiences, with reports of each model enhancement contributing to higher user retention and deeper engagement.
From its inception, MiniMax has demonstrated a commitment to independent decision-making in its business focus, technical direction, and AGI development strategy. The company has avoided trend-following and focused on addressing complex challenges through non-conventional methods.
The large model industry has now entered its second half. The low-hanging fruits have been picked clean, leaving little room for success through imitation or following trends.
Instead, markets can now only be gained and sustained through continued innovation. To stay ahead, both new ideas and a willingness to pursue less conventional paths are quintessential.
KrASIA Connection features translated and adapted content that was originally published by 36Kr. This article was written by Xiao Xi for 36Kr.