An intriguing development surfaced early this year: Agibot, a rising star in embodied intelligence, unveiled what it calls a “data acquisition center” in Shanghai. Notably, the company’s CTO is Peng Zhihui, a prominent Bilibili creator well-regarded in the tech community.
But what exactly is a data acquisition center? Why was it established? How does it function? These questions sparked curiosity, prompting 36Kr to take a closer look at the facility.
Initially, the term “data acquisition center” conjured a predictable image: a dimly lit room filled with server racks, where weary programmers with dark circles hunched over their keyboards. Reality, however, turned out to be far more fascinating.
When 36Kr arrived at Agibot’s data acquisition center in Pudong, Shanghai, the experience was akin to stepping onto a Star Wars set. Spanning a 3,000-square-meter facility, the center is a meticulously designed space where robots interact with environments crafted to mimic real-life scenarios.
In one room resembling a bedroom, robots diligently practiced folding clothes. In another, designed as a dining area, they carefully arranged utensils at a table with precision. The kitchen setup showcased robots plating dishes with a steady hand, while at a simulated supermarket checkout counter, robots were seen operating barcode scanners in one hand while balancing products in the other.
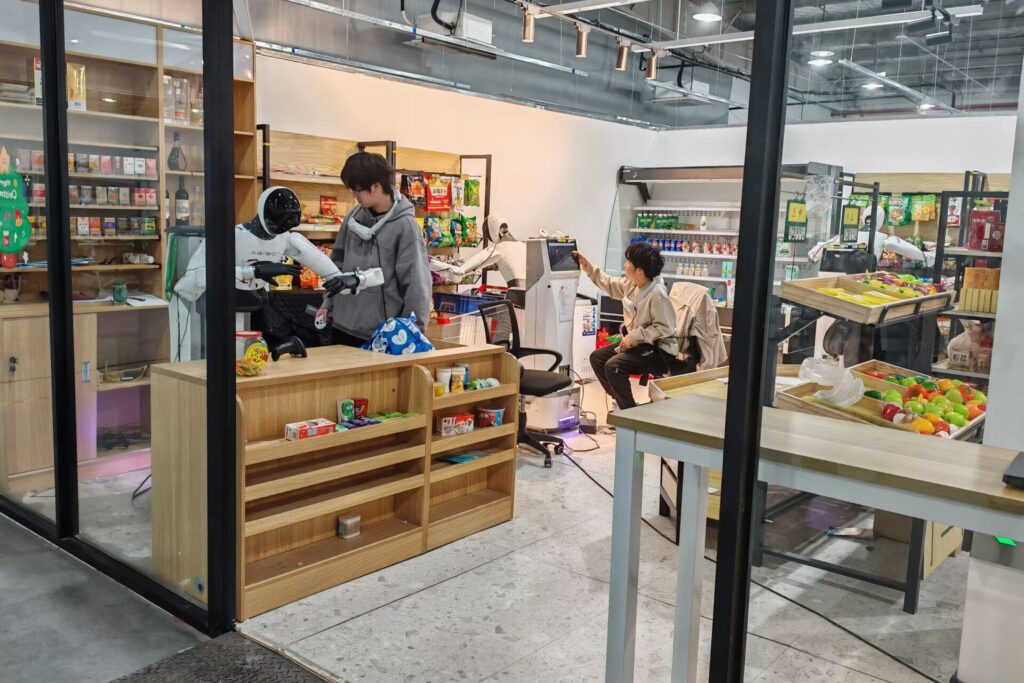
Following the tour, 36Kr spoke with Yao Maoqing, who oversees the data acquisition center and Agibot’s embodied intelligence product line. Yao also serves as executive president of Agibot’s research development.
Yao’s background includes work on perception algorithms and end-to-end (E2E) large models at companies like Waymo and Nio. Drawing on this expertise, Yao explained that every action a robot performs generates data. This data is uploaded from the robot’s mainframe to the cloud, where Agibot’s team uses it to train large models. These models, in turn, enable robots to truly master complex tasks, such as brewing coffee or ironing clothes.
To make this learning process more efficient, Agibot assigns an “instructor” to each robot. These instructors are skilled data collectors, typically young professionals with exceptional physical coordination and precision. They play a vital role in teaching robots specific actions by providing hands-on guidance.
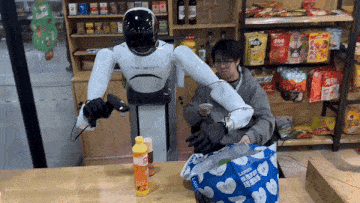
Using handheld devices, the instructors guide robots step-by-step through tasks like grabbing, holding, and placing objects. In some cases, they wear virtual reality headsets to help the robots replicate human movements with greater accuracy.
Currently, the data acquisition center deploys nearly 100 robots, which collectively generate 30,000–50,000 data points every day.
To speed up robots’ mastery of skills across various environments, the center focuses on simulating five major scenarios: home, retail, service, dining, and industrial settings.
In the simulated retail environment, for instance, shelves are stocked with snacks, wine, cigarettes, and labeled prices for fruits and vegetables, creating a lifelike training ground. Elsewhere, robots practice simpler tasks, such as folding clothes at mock workstations.
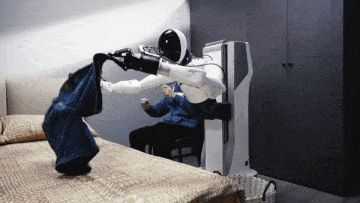
Agibot plans to expand the facility by 1,000 square meters, allowing for the addition of more simulated scenarios and customized environments tailored to client needs. This approach is uncommon in the robotics industry, sparking a key question: Why did Agibot invest heavily in such a facility, and how did they build it so quickly?
For most startups, constructing a data acquisition center is a high-risk move, but Agibot embraced the challenge and managed to complete the center in just over a month. The motivation? A glaring industry gap: the lack of high-quality training data.
In June 2024, Agibot committed to developing a large model for embodied intelligence, a process requiring vast amounts of training data. Yao explained that robots typically need hundreds of data points to master a single skill, particularly repetitive tasks like brewing coffee or ironing clothes. Initially, the company explored open-source datasets, but it found these resources unsuitable.
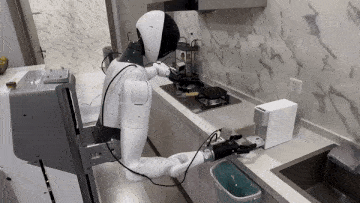
“The quality and consistency just weren’t there,” Yao told 36Kr. “Data collected by different companies, using robots with varying designs and sensors, often couldn’t be applied across the board. For instance, data from a six-axis robotic arm isn’t compatible with a seven-axis flexible robot, which weakens training outcomes and lowers efficiency.”
The limitations of existing datasets made Agibot realize it needed a proprietary solution. Earlier training efforts using a few thousand data points enabled robots to perform certain actions but highlighted a critical shortcoming: lack of generalization. Robots struggled to adapt to variations in objects’ types, colors, or lighting conditions, making their skills unreliable in real-world scenarios.
Recognizing the necessity of large-scale, high-quality data, Agibot built its center to ensure a steady stream of reliable data. In just two months of operation, the facility has generated over one million real-world data points and supports more than 1,000 distinct tasks. Each task produces hundreds or even thousands of data points.
“We’ll have tens of millions of data points in the near future,” Yao said, smiling.
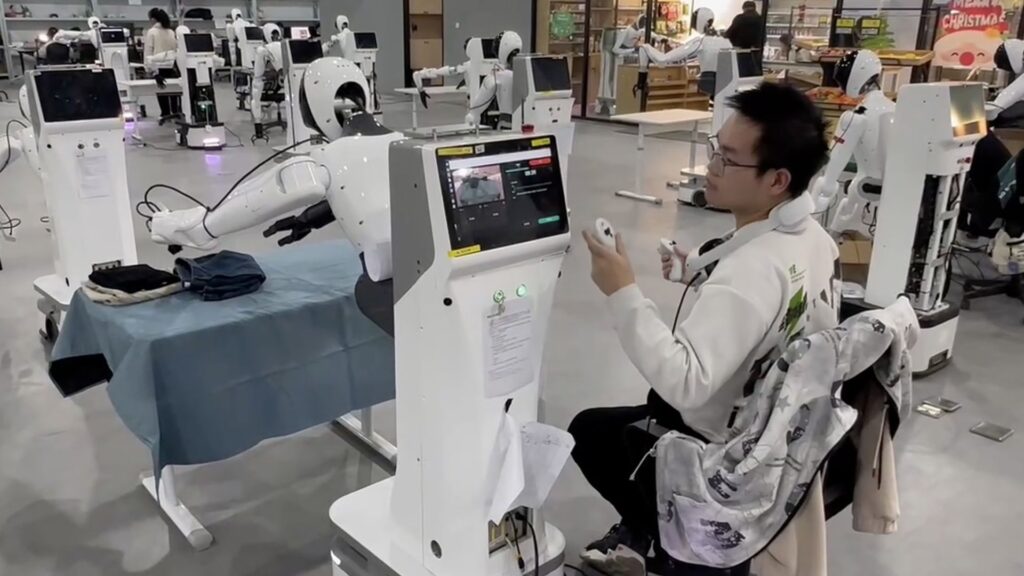
The “scaling law” of robotics
While choreographing repetitive robot actions to collect data points, Agibot stumbled upon unexpected breakthroughs. For instance, robots could adjust the amount of water poured into a glass without additional training. With just a few dozen demonstrations, they learned to fold pants.
This capability reflects the kind of robot Agibot aims to build—one that can autonomously interpret human instructions, adapt to external environments, and excel in complex, dynamic scenarios.
For decades, robotic control systems relied on predefined rules. Programmers painstakingly input detailed instructions for specific tasks under narrowly defined conditions. While effective in static environments, these systems faltered when faced with unpredictable real-world situations where pre-programmed responses were insufficient.
The advent of large models has upended this paradigm. These models enable robots to interpret the world and understand human instructions in ways that were previously impossible. Agibot is now developing robots powered by E2E large models, which offer both adaptability and rapid response capabilities.
Traditionally, robots perform tasks in three modular steps:
- Perceiving the external environment.
- Making decisions based on the input.
- Controlling physical actions to execute tasks.
However, these modular processes are prone to distortions at each stage, impacting overall performance. E2E large models bypass these steps entirely, mimicking human behavior instead of relying on rigid measurements or pre-programmed rules. For instance, a robot using an E2E model might overtake a car without calculating precise distances—similar to how humans rely on instinct and context.
Agibot envisions robots capable of handling complex commands, such as retrieving a phone from another room or fetching a bag of chips from the refrigerator. These tasks demand not only the ability to understand instructions but also to identify objects, navigate spaces, and execute multi-step processes seamlessly.
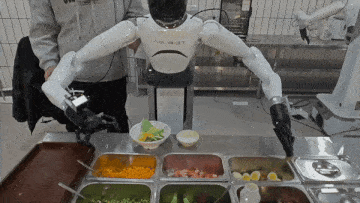
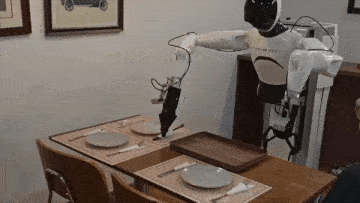
However, achieving this vision is no small feat. Yao Maoqing emphasized the critical role of feeding continuous streams of data into large models. The more data these models ingest, the closer they come to human-level performance in specific scenarios. Yet, scaling robotics to this level will require tens of millions—if not billions—of data points. The “scaling law” of robotics is still a work in progress, far from being fully realized.
Ultimately, the advancement of robotics depends on integrating hardware and software—prioritizing one at the expense of the other is unlikely to yield meaningful progress.
In the US, where hardware costs are high, most robotics startups prioritize algorithm development while outsourcing hardware. China, however, benefits from a robust and cost-effective supply chain. By combining advanced algorithms with self-developed hardware, Chinese robotics companies can iterate more quickly and efficiently.
Yao highlighted that China’s robotics industry is now on par with the US. “Labor costs in the US are ten times higher than in China, and US companies still rely on Chinese suppliers for components,” he said.
This cost advantage, coupled with faster development cycles, has allowed Agibot to expand its capacity for scene simulation and data collection. Technologies that US robotics companies might find cost-prohibitive are increasingly attainable in Agibot’s facility, where streams of data are steadily transforming futuristic ideas into practical realities.
KrASIA Connection features translated and adapted content that was originally published by 36Kr. This article was written by Tian Zhe for 36Kr.